Synthetic Data: What is it and Why it matters
- GPaolo Neri
- Mar 12
- 4 min read

Have you ever wondered how AI can solve real-world problems without touching a single piece of real data?
Welcome to synthetic data—the invisible player quietly rewriting the rules. It’s fake data, built by algorithms, yet it’s driving real progress in business, daily life, and beyond.
Definition: Synthetic data is artificially generated data used as a supplement or alternative to real-world data, which is often constrained by cost, availability, or privacy regulations (Gartner)
Many people don’t know about it yet, but this invisible force is already shaping the world around us. So, what exactly is synthetic data, and why does it matter?
Picture it as a substitute for real data. When actual info—like your shopping history or health records—is too tricky to use, too private, or just not enough, AI steps in to create artificial datasets that mirror reality without copying it. A 2023 Gartner report predicts, “By 2030, synthetic data will overtake real data in AI training”—a bold sign this isn’t just a passing trend.
AI companies use synthetic data beyond privacy concerns to overcome limited real-world data, ensuring they have enough variety for training robust models. It also helps simulate rare or hard-to-find scenarios, like extreme weather or medical cases, which real data might lack. Additionally, it speeds up development by avoiding the time-consuming process of collecting and labeling real data. Finally, it reduces costs associated with acquiring and processing large datasets.
Benefits of Synthetic Data
Enhanced Privacy and Security: Synthetic data protects privacy by not containing personal information, making it ideal for compliance with regulations like GDPR.
Accessibility and Cost Efficiency: It offers unlimited data generation, reducing costs associated with real data collection and storage.
Bias Reduction: Synthetic data can mitigate biases present in real-world data, ensuring more equitable AI outcome.
Innovation and Experimentation: Enables testing of new scenarios and hypotheses without risking real systems or users.
Pros | Cons |
Overcomes limited real-world data, ensuring variety for robust models. | May lack the richness and nuances of real-world data, reducing accuracy. |
Simulates rare or hard-to-find scenarios, like extreme weather or medical cases. | Requires expertise to create high-quality, realistic synthetic data. |
Speeds up development by avoiding time-consuming data collection/labeling. | May inadvertently introduce biases if poorly designed. |
Reduces costs associated with acquiring and processing large datasets. | Some industries may face challenges validating synthetic data for compliance purposes. |
Applications across industries
Take car insurance, for instance. An insurer wants to predict accident risks, but real crash data is a nightmare—privacy walls, gaps, etc. Synthetic data saves the day. AI creates a fake city of drivers—some wild, some careful—complete with imaginary crashes, weather twists, and road issues. The insurer fine-tunes its models in this simulated environment, developing improved policies without affecting your real-life accident. The payoff? Smarter pricing, safer streets, and your privacy stays intact. That’s the phantom engine doing its thing.
Bank fraud, another example, is a relatively rare event in the real world, meaning that collecting sufficient data points to train AI systems to flag fraudulent transactions is difficult without recourse to synthetic data.
In financial markets, AI is frequently used to gauge business outcomes using synthetic scenarios such as supply chain disruption, inflationary factors and stock market volatility. What’s more, synthetic data can be used to train AI to react to customer activities analysing shopping patterns, online activities and more. Here, synthetic data is created from actual data but sanitized to remove any potential personal information or unintentional bias.
Businesses are jumping on this—using it to prototype apps or train chatbots without real user details. In everyday life, it’s sharpening your gadgets and apps behind the scenes. Think of a fitness tracker that learns from fake joggers before fixing your pace. It sidesteps data shortages and keeps regulators off your back, all while pushing AI to new heights. For companies, it’s a goldmine; for us, it’s the unseen power making tech feel effortless.
Future trends and importance
While synthetic data is growing fast—potentially 30% of AI training data in 2025 compared to real data—it’s not yet the dominant force. Let’s say the total usable real data for AI training in 2025 is around 1 exabyte (1 trillion megabytes, including: billions of webpages, books, and user-generated content), a conservative estimate given the scale of global data. Its magnitude varies by field, from a small part in data-rich areas to the primary source in simulation-heavy ones. By 2030, Gartner’s prediction is that synthetic data will overtake real data in AI training, but for now, real data still holds the lead in most cases.
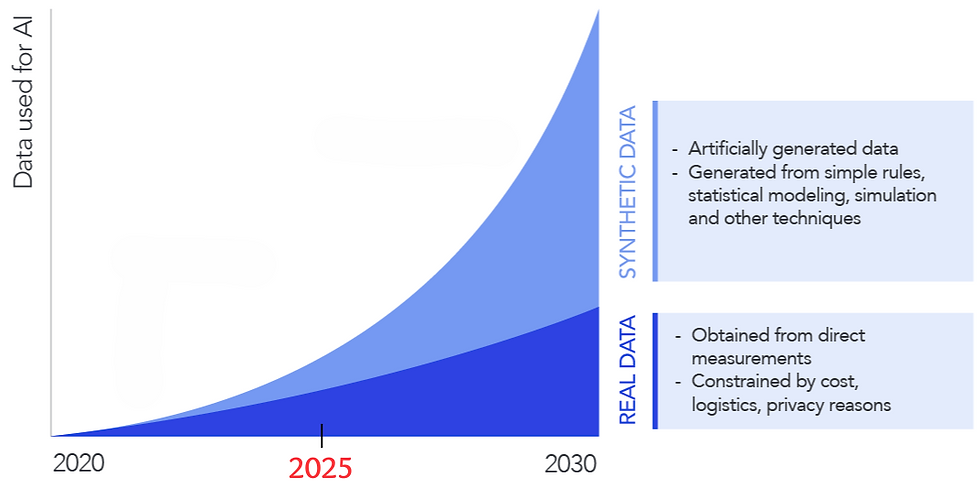
But here’s where it gets wild: this invisible force might change reality in the future. If it gets too good, will we rely on it more than the real thing? Could it fuzz the line between truth and invention? It’s not just a tool—it’s a sign of what’s coming, where fake stuff tackles real challenges. Right now, it’s the quiet force behind sharper AI, from insurance tweaks to self-driving cars.
Next time your app seems spot-on, performing exceptionally well… tip your hat to synthetic data! —this invisible player creating successes you didn’t expect. Where’s this quiet revolution headed? Stay tuned: it’s only warming up.
Sources:
GARTNER: https://www.gartner.com/en/newsroom/press-releases/2022-06-22-is-synthetic-data-the-future-of-ai
GLOBALDATA: https://www.globaldata.com/store/report/synthetic-data-master-key-to-ai-future-trend-analysis/
Comments